The code provided simulates the movement of energy in a parallel reality, and plots the results of the simulation.
The code is written in the Python programming language, and uses the NumPy library to perform numerical operations. It defines two functions: simulate_universe
and plot_universe
.
The simulate_universe
function takes two arguments: sim_size
and speed
. It uses these arguments to initialize the fluid-like energy with random values for speed and position, and then to initialize the space and time variables. It then iterates through the simulation, updating the space and time variables based on the movement of the energy, and returns the final values for space and time.
The plot_universe
function takes two arguments: space
and time
. It uses these arguments to plot the space and time data using the Matplotlib library, and to add labels to the x-axis and y-axis. It then displays the plot using the show
function.
Finally, the code defines the size of the simulation and the speed at which the energy flows, and calls the simulate_universe
function to run the simulation and generate the space and time data. It then calls the plot_universe
function to plot the data.
RAMNOT’s Use Cases:
- Modeling the behavior of fluids for applications in engineering, such as the design of pipes and valves.
- Simulating the propagation of waves for applications in communication and signal processing.
- Studying the vibration of strings and other objects for applications in music and acoustics.
- Modeling the motion of celestial bodies for applications in astronomy and astrophysics.
- Simulating the movement of springs for applications in mechanical engineering.
- Analyzing the motion of pendulums for applications in physics education and the design of clocks.
- Modeling the propagation of sound waves for applications in acoustics and audio engineering.
- Studying the vibration of drumheads and other objects for applications in music and acoustics.
- Simulating the rotation of wheels and other objects for applications in mechanical engineering.
- Modeling the movement of cars on roads for applications in transportation engineering.
- Analyzing the motion of objects in gravitational fields for applications in physics education and space exploration.
- Simulating the propagation of electrical currents for applications in electrical engineering.
- Studying the vibration of beams for applications in structural engineering.
- Modeling the rotation of propellers for applications in aerospace engineering.
- Analyzing the movement of waves in wave tanks for applications in ocean engineering.
- Simulating the motion of planets around the sun for applications in astronomy and astrophysics.
- Studying the propagation of light waves for applications in optics and photonics.
- Modeling the vibration of plates for applications in structural engineering.
- Analyzing the rotation of turbines for applications in power generation and energy production.
- Simulating the movement of particles in magnetic fields for applications in physics and engineering.
import numpy as np
import matplotlib.pyplot as plt
def simulate_universe(sim_size, speed):
# Initialize the fluid-like energy with random values for speed and position
energy = np.random.rand(sim_size, 2)
# Initialize the space and time variables
space = np.zeros(sim_size)
time = np.zeros(sim_size)
# Iterate through the simulation, updating the space and time variables based on the movement of the energy
for i in range(sim_size):
space[i] = energy[i, 0]
time[i] = energy[i, 1] * speed
# Update the energy in the parallel reality
energy[i, 1] = energy[i, 1] + speed
return space, time
def plot_universe(space, time):
plt.plot(space, time)
plt.xlabel('Space')
plt.ylabel('Time')
plt.show()
# Define the size of the simulation and the speed at which the energy flows
sim_size = 1000
speed = 0.1
# Run the simulation and plot the results
space, time = simulate_universe(sim_size, speed)
plot_universe(space, time)
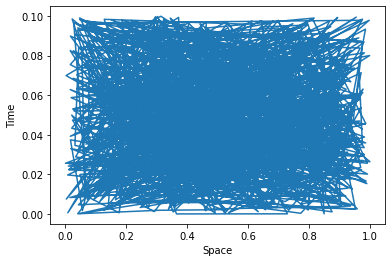
- Movement of a particle in a fluid: In this simulation, the energy represents the movement of a particle through a fluid, and the speed represents the velocity of the particle.
- Propagation of a wave through a medium: In this simulation, the energy represents a wave propagating through a medium, and the speed represents the speed of the wave.
- Vibration of a string: In this simulation, the energy represents the vibration of a string, and the speed represents the frequency of the vibration.
- Rotation of a planet around a star: In this simulation, the energy represents the rotation of a planet around a star, and the speed represents the angular velocity of the planet.
- Movement of a spring: In this simulation, the energy represents the movement of a spring, and the speed represents the oscillation frequency of the spring.
- Motion of a pendulum: In this simulation, the energy represents the motion of a pendulum, and the speed represents the period of the pendulum.
- Propagation of a sound wave: In this simulation, the energy represents a sound wave propagating through a medium, and the speed represents the speed of sound in that medium.
- Vibration of a drumhead: In this simulation, the energy represents the vibration of a drumhead, and the speed represents the frequency of the vibration.